Communication is key to intelligence, it allows sharing of data and computational resources, and enables cooperation and coordination in multi-agent environments. However, in the machine learning literature, communication links are often modelled as ideal error-free bit pipes, which is far from reality; communication channels suffer from noise, power loss, and interference, and are prone to time-variations. In wireless networks error-free communication is a rarity rather than a norm. Currently, distributed machine learning and noisy communication problems are treated separately, and error correction and interference management techniques are employed independently from the underlying learning task. In this talk, systematically going through inference, training, and reinforcement learning problems, I will argue that a strict separation between learning and communication can be highly suboptimal, and I will propose a joint learning and communication framework, illustrating its benefits on various distributed learning problems.
Prof. Deniz Gunduz Professor at Imperial College Londonemail: Questo indirizzo email è protetto dagli spambots. È necessario abilitare JavaScript per vederlo.
Department of Information Processing in the Electrical and Electronic Engineering
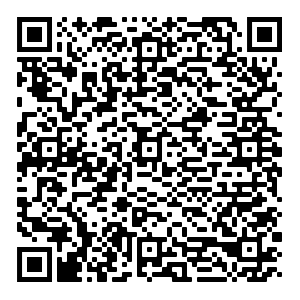