Federated Learning (FL) refers to distributed protocols that avoid direct raw data exchange among the participating devices while training for a common learning task. This way, FL can potentially reduce the information on the local data sets that is leaked via communications. This lecture covers three key, and often overlooked, requirements of FL: ensuring privacy, guaranteeing trustworthy, well calibrated, decisions, and improving on-device energy efficiency. When FL is implemented in wireless systems via uncoded transmission, it is first argued and proved that the channel noise can directly act as a privacy-inducing mechanism. Ensuring trustworthy decisions requires quantifying uncertainty, which can be done in a principled way by moving from the conventional frequentist learning framework to a Bayesian formulation. The lecture will review federated Bayesian learning and introduce Distributed Stein Variational Gradient Descent (DSVGD), a non-parametric generalized Bayesian inference framework for federated learning. Finally, the talk will introduce Spiking Neural Networks (SNNs) as biologically inspired machine learning models that build on dynamic neuronal models processing binary and sparse spiking signals in an event-driven, online, fashion. SNNs can be implemented on neuromorphic computing platforms that are emerging as energy-efficient co-processors for learning and inference. FL for the distributed training of SNNs is presented and initial results are presented.The seminar focus on a new perspective to overcome this drawbacks by combining the holographic technology with Artificial Intelligence and 5G connection to speed up the computational processing and permits fast connection with central laboratory or hospital
Prof. Osvaldo Simeoneemail: osvaldo.simeone_at_kcl.ac.uk
Professor of Information Engineering King’s College London and Director of King's Communications, Information Processing & Learning (KCLIP) lab
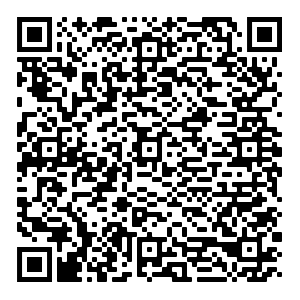